Introduction
In the clinical follow-up of metastatic breast cancer patients, semi-automatic measurements are performed on 18FDG PET/CT images to monitor the evolution of the main metastatic sites. Apart from being time-consuming and prone to subjective approximations, semi-automatic tools cannot distinguish between cancerous regions and active organs presenting a high 18FDG uptake.
In the context of the Epicure project, we developed and compared fully automatic deep learning-based methods segmenting the main active organs (brain, heart, bladder), from full-body PET images.
Methods
We combined deep learning-based approaches with superpixel segmentation methods. In particular, we integrated a superpixel SLIC segmentation algorithm1 at different depths of a convolutional neural network2, i.e. as input U-Net-SP-Input and within the network loss U-Net-SP-Loss.
Superpixels reduce the resolution of the images, keeping the boundaries of the larger target organs sharp while the lesions, mostly smaller, are blurred. Results are compared with a deep learning segmentation network.
The methods are cross-validated on full-body PET images of 36 acquisitions from the ongoing EPICUREseinmeta study. The similarity between the manually defined ground truth masks of the organs and the results is evaluated with the Dice score. The ground truth masks were delineated on the Keosys Viewer. Moreover, to be sure that no lesions were detected by these methods, we counted the number of voxels classified as belonging to an active organ while in fact they belonged to a lesion.
Results
Although the methods present similar high Dice scores (0.96 ± 0.006), those using superpixels present a higher precision (on average 6, 16, and 27 selected voxels belonging to a tumor, for the CNN integrating superpixels in input, in optimization and not using them, respectively). Moreover, looking at the following images, the U-Net method, which does not integrate super pixel information, segmented tumor parts instead of active organs. The other two U-Net methods correctly segment the active organs, providing the results we were hoping to obtain.
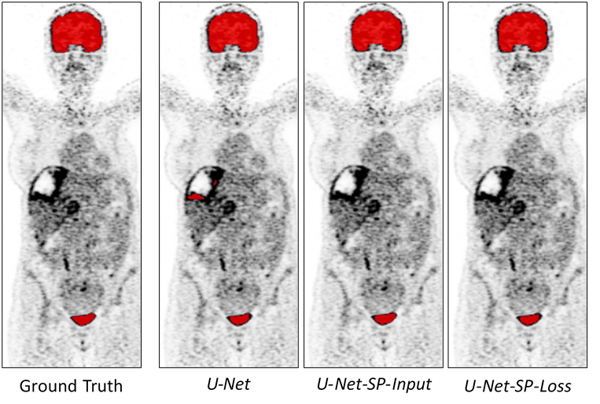
Conclusion
Combining deep learning with superpixels allowed us to segment organs presenting a high 18FDG uptake on PET images without selecting cancerous lesions. This improves the precision of the semi-automatic tools monitoring the evolution of breast cancer metastasis.
Bibliography
[1] R. Achanta, A. Shaji, K. Smith, A. Lucchi, P. Fua, and S. Süsstrunk, “SLIC superpixels compared to state-of-the-art superpixel methods,” IEEE Trans. Pattern Anal. Mach. Intell., vol. 34, no. 11, pp. 2274–2281, 2012.
[2] F. Isensee et al., “nnU-Net: Self-adapting Framework for U-Net-Based Medical Image Segmentation,” Inform. aktuell, p. 22, 2019.