At the beginning of the year, an article in Nature Medicine was published that provided a timely overview of the potential and pitfalls of the rapidly growing phenomenon of incorporating artificial intelligence (AI) in medicine.
A highly notable item in the article was the increasing rate at which the US FDA was approving AI algorithms for image interpretation (Table 1): from just 2 in 2017 to 12 in 2018.
At Keosys, we recognize that it will only be a matter a time before AI will profoundly affect the clinical trial arena. Here, we briefly discuss AI and the opportunities of using this powerful tool in medical imaging.
What is AI?
AI encompasses a broad range of fields and techniques, and its definition and applications have markedly evolved from when the term was first formulated in the 1950s. At first, the aim was to develop machines that can replicate human intelligence. Now, most AI efforts focus on applying human reasoning (i.e., problem solving) to guide efforts to provide improved services or products.
Regarding AI and medical imaging, machine learning and deep learning are the subtypes of AI that are of most interest (Fig. 1). In machine learning, a computer is given data to learn from without explicit programing. Delving down the hierarchy, deep learning is one of the techniques used for implementing machine learning. The big advantage of deep learning is that the system can automatically and independently learn to associate low-level features in an image (e.g., texture, lines or edges) and consolidate them to higher-level features (e.g., shapes, tumors or organs) in the subsequent layers.
Simply put, algorithms enable computers to learn to identify patterns in images and to predict an output. The difference between machine and deep learning is how they achieve their goals. In short, standard machine learning algorithms require human interactions to extract informative features, whereas deep learning solves the problem from end to end (for more details of the differences between machine and deep learning see here) without human interactions.
But why develop such algorithms? High-throughput, unbiased and accurate analysis is a prime goal. For example, the FDA-approved LVO Stroke Platform from Viz.ai can identify a large vessel occlusion (LVO) and alert the relevant specialist with 90% sensitivity and specificity and a median time of scan to notification of <6 minutes.
That is, the platform analyzes CT images of the brain and automatically notifies a specialist within the same time a radiographer is conducting a review of the images. Earlier notification of a specialist can mean earlier interventions—a crucial factor for treatment success in stroke.
Meanwhile, another notable FDA approval last year was IDx-DR, which is used to detect diabetic retinopathy. IDx-DR was the first approved AI device that provides a screening decision without requiring a clinician to also interpret the image or results. Consequently, healthcare providers who may not normally be involved in eye care can diagnose this condition.
Both these platforms provide a snapshot of the potential of AI in medical imaging: speed up diagnoses and widen the personnel who can ‘interpret’ images.
AI and Imaging in Clinical Trials
The LVO Stroke Platform and IDx-DR both use deep learning algorithms, and this subtype of AI is the future of AI in medical imaging. Indeed, a survey of deep learning methods developed for medical image analyses reviewed more than over 300 contributions to the field, most of which were published in recent years. However, the promise of AI must be tempered by the knowledge that the algorithms are only as good as the data that they are trained on.
Clinical trials are a rich resource for developing and applying AI, as the vast generated datasets of images (plus the accompanying metadata) provide valuable information for training and validating algorithms. Indeed, applications such as segmentation (identifying structures of interest such as organs, lesions and tumors), detection, registration and classification (e.g., whether a lesion is benign or malignant) are the focus of most research efforts to improve accuracy (e.g., see the challenges at the Grand Challenges in Biomedical Image Analysis website).
Once validated, such tools could be deployed for redundant and repetitive delineation tasks (e.g., see Fig. 2), releasing the radiologist to perform higher-level tasks. Consequently, clinical trials could ultimately require less time and money, as repetitive, image-related tasks will be performed in an automated manner. At Keosys, we know that deep learning is already working quite well for selected medical image processing applications (e.g., segmentation). Therefore, we plan to introduce deep learning-based methods in our viewer in the next 2 years.
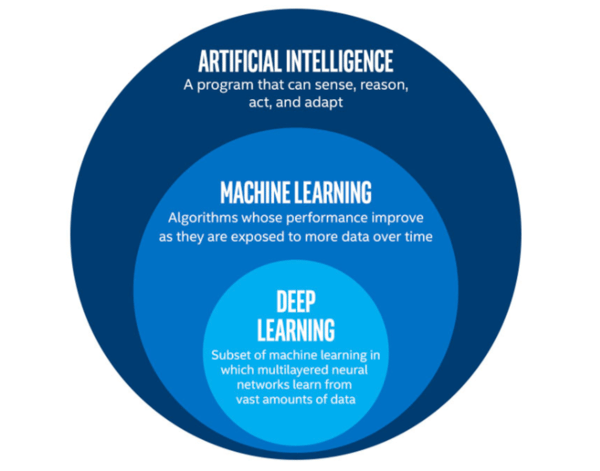
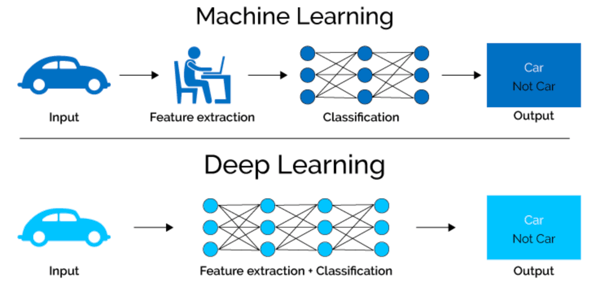
Top: AI is an umbrella term that encompasses machine learning and deep learning. Bottom: In machine learning, features are identified by an expert to make patterns more visible to learning algorithms to work. In deep learning, algorithms learn high-level features from data in a stepwise manner.
Sources: Singh, S. Towards Data Science (27 May 2018); Mahapatra, S. Towards Data Science (21 Mar 2018).
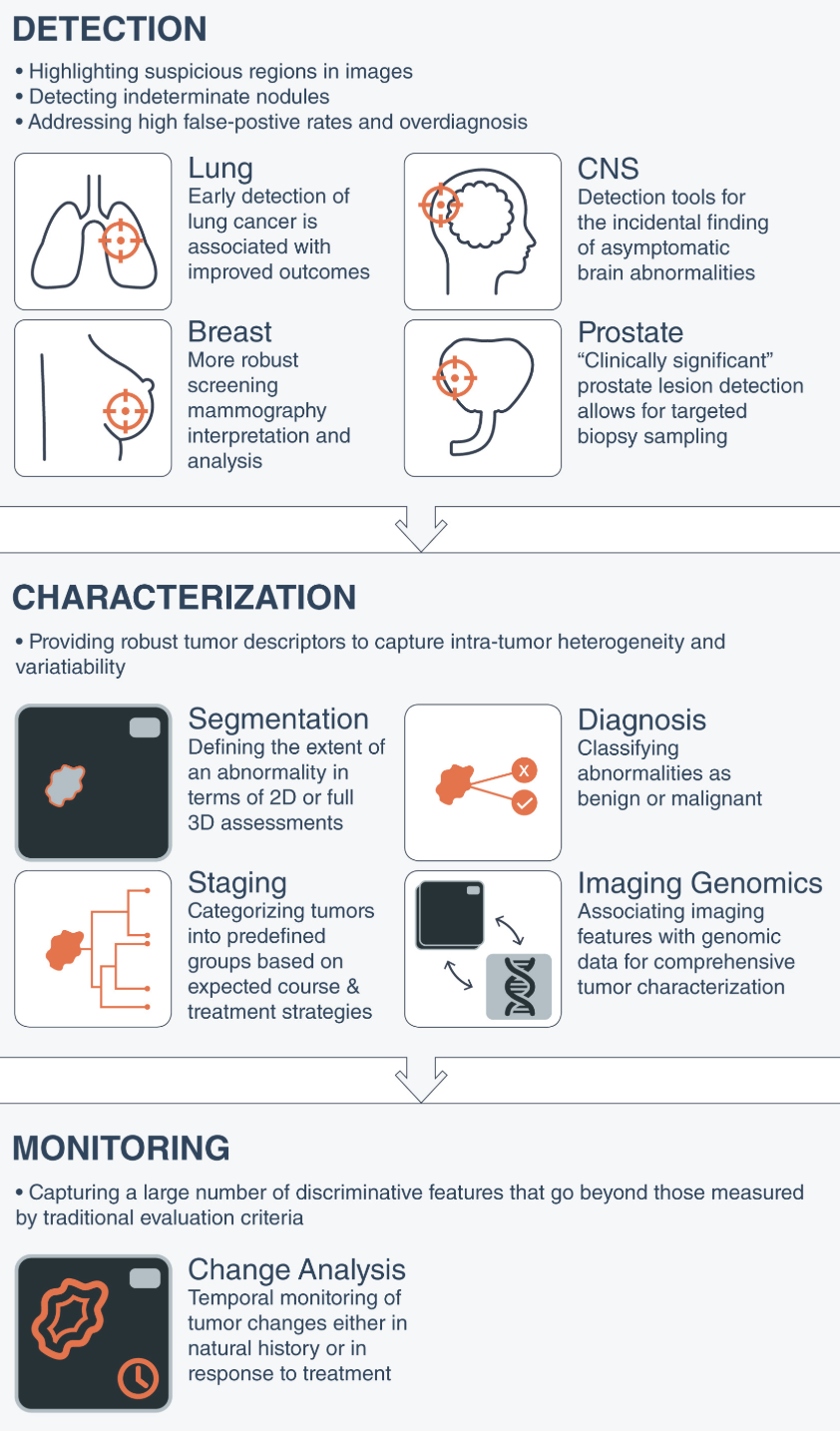
Source: CA Cancer J. Clin. 69, 127-157 (2019)