Medical imaging is used to detect tumors and measure changes in their size over time. The reproducibility and accuracy of imaging-based tumor measurements are important as clinical decisions and drug efficacy determinations are often based on the assumption that a change in imaging measurements reflects a true change in tumor size.
The interpretation of a medical image is known as the read, and the expert performing the read is known as the reader (or observer). Reading an image is inherently subjective, and as such there is variability in the reads. There are two main types of reader variability (also known as observer variability):
- Intra-reader variability: measurement differences from the same reader, which usually stem from the difficulty of the imaging case or reader interpretation drift (i.e., deviation from study-specific image interpretation criteria on which readers have been trained)
- Inter-reader variability: measurement differences between two or more readers, usually due to readers’ skill.
Reader variability can in turn increase the variability of clinical trial data and lead to misinterpretations of cancer drug efficacy. Differential ways of acquiring the image, the level of reader training and the information that is available to the reader all contribute to reader variability.
Here, we outline six ways in which reader variability can be reduced.
1. Standardized Image Acquisition
Ensuring that images are collected in a standard way is achieved through the use of imaging acquisition guidelines (IAG) and an imaging review charter (IRC). The IAG outlines standards — related to equipment, imaging drug, radiation exposure etc. — needed to get consistent and reproducible image acquisition across each investigator site. The IRC describes the steps needed to maximize data integrity, such as the correct imaging workflow, training and other operational steps.
2. Uniformity of Information
A central read, where the image interpretation takes place away from the study site using blinded readers (who are unaware of e.g. patient characteristics) ensures that the information given to each reader is uniform. This will reduce reader bias and is likely to produce high quality data due to the controlled and transparent setting.
3. Standardized Reader Training
Readers should be qualified based on the indication and the response criteria specified in the trial protocol. A standardized training program, including interpretation methods and criteria application, for all readers involved in a specific study will help mitigate reader discordance. Several publications have shown that dedicated training programs improve the accuracy of image interpretations of cancer.
4. Adjudicator to Manage Discordance
Large therapeutic trials typically use more than one reader. Discordance between two readers in a trial should be adjudicated by a third reader who has the ability to review the outcomes and select what they understand to be the most correct assessment. Adjudication is triggered when there is a discrepancy on the main points of assessment (progressive disease assessment, best overall response assessment…). Points of adjudication are identified by the sponsor and/or a specialist imaging company prior the start of the trial.
5. Perfomance Monitoring
To keep variability as low as possible, reader-specific performance, including adjudication rates and inter- and intra-reader variability, should be monitored throughout the trial. This will identify potential issues early and mitigate unnecessary discordance. Commonly used statistical measures to capture this data include Kappa statistics (intra-reader agreement) and concordance correlation coefficients (inter-reader reliability).
6. Retraining and Calibration Meetings
If cases associated with substantial discordance are identified, then re-training of readers in the best practice for that specific trial will mitigate future discordance. Calibration meetings might be necessary, where readers come together to discuss difficult cases to and achieve agreement on a 'consensus read'.
The Future: Removing Human Error
Image interpretation is inherently subject to human error. Advances in analytical technology and artificial intelligence offer ways to remove this error. Several studies have shown that technology improves standardization. One study found that computer-assisted measurements reduced inter-reader variability by one third to one half compared to manual measurements.
Artificial intelligence can aid:
- Tumor detection Deep learning algorithms can automatically identify tumors or suspicious lesions in images. This can enable earlier diagnosis as lesions that might not be easily spotted by humans can be identified.
- Lesion tracking Technology-aided tools that track a lesion over time are particularly exciting. They ensure that changes in tumor size between reads can be easily monitored and reader variability is minimized.
You can read more about the role of AI in medical imaging in our blog post here.
Being aware of how and when variability can arise in a clinical trial is important. There can be different sources of variability depending on the specificities of the trial. A specialist imaging company, such as Keosys, will share their expertise and provide advice on the best methods to implement to reduce variability in your clinical trial.
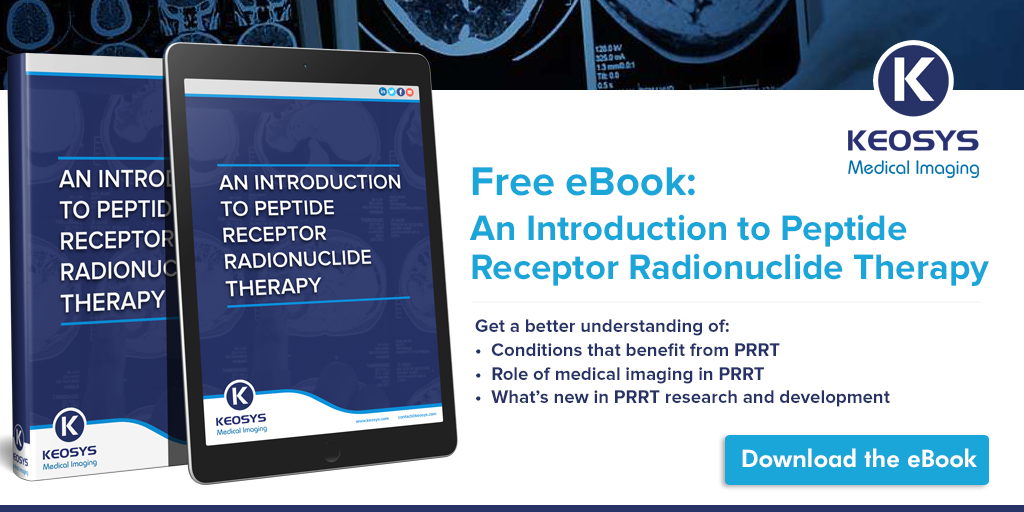